In recent literature, it has been shown that the
number of steps in a sequential quadratic programming algorithm for a non-linear model predictive control (NMPC) problem can be greatly reduced by a parallel shooting method. The
efficiency of such a parallel shooting method further depends
on how the algorithm is implemented on parallel computing
platforms such as Graphics Processing Units (GPUs). The
GPU implementation should consider the degree of parallelism
necessary for higher time efficiency as well as the hardware
resource consumption/limitation at the GPU for a given problem
size. In this paper, we present a multilevel parallel GPU
implementation for sequential quadratic programming and an
(Alternating Direction Method of Multipliers) ADMM solver.
First, we introduce a GPU implementation enabling parallel
computing of many quadratic programs (QPs) by functional
parallelism. Next, we parallelize each QP solver using data
parallelism of basic linear matrix operations. We show that the
proposed GPU implementation greatly scales with the degree
of parallelism in the parallel shooting method. Further, we
show how a GPU implementation can be configured for a given
problem size avoiding resource overprovisioning. Read more here: https://doi.org/10.23919/ECC64448.2024.10590998
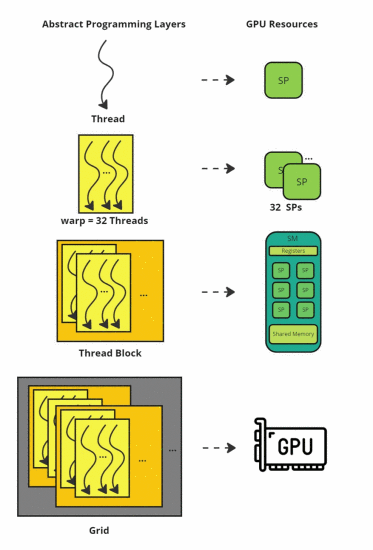