Data-driven predictive control (DPC) has gained an increased interest as an alternative to model predictive control in recent years, since it requires less system knowledge for implementation and reliable data is commonly available in smart engineering systems. Several data-driven predictive control algorithms have been developed recently, which largely follow similar approaches, but with specific formulations and tuning parameters. This review aims to provide a structured and accessible guide on linear data-driven predictive control methods and practices for people in both academia and the industry seeking to approach and explore this field. To do so, we first discuss standard methods, such as subspace predictive control (SPC), and data-enabled predictive control (DeePC), but we also include newer hybrid approaches to DPC, such as gamma–data-driven predictive control and generalized data-driven predictive control. For all presented data-driven predictive controllers we provide a detailed analysis regarding the underlying theory, implementation details and design guidelines, including an overview of methods to guarantee closed-loop stability and promising extensions towards handling nonlinear systems. The performance of the reviewed DPC approaches is compared via simulations on two benchmark examples from the literature, allowing us to provide a comprehensive overview of the different techniques in the presence of noisy data. Read more here: https://www.sciencedirect.com/science/article/pii/S1367578823000780
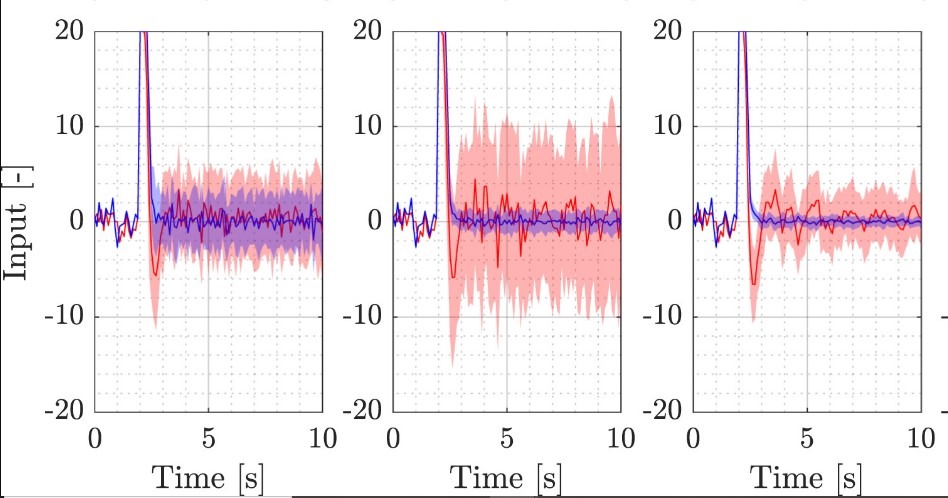